Revolutionizing Diagnostics: How AI is Transforming Medical Laboratory Testinge here
From Data Overload to Precision Insights — The AI-Powered Lab of Tomorrow
Why This Matters
Artificial Intelligence (AI) is no longer science fiction. In medical laboratories, AI tools are reducing human error, speeding up diagnoses, and unlocking patterns in vast datasets that humans alone cannot process. By 2025, the global AI diagnostics market is projected to exceed $4.7 billion (WHO, 2023), making this a critical topic for lab professionals.
Image Suggestion:
- A split-screen graphic showing a traditional lab vs. an AI-integrated lab (e.g., robots handling samples, AI dashboards).
Key Applications of AI in Labs
1. Automated Image Analysis
AI algorithms analyze microscopic images (e.g., blood smears, tissue biopsies) with >95% accuracy.
- Example: PathAI’s platform detects cancerous cells in histopathology slides faster than manual review.
- Simple Explanation: AI “learns” from thousands of labeled images to recognize patterns (e.g., abnormal cell shapes).

2. Predictive Diagnostics
Machine learning (ML) models predict diseases like sepsis or heart failure hours earlier by analyzing lab results, vital signs, and patient history.
- Example: Johns Hopkins’ AI model predicts sepsis 12 hours before symptoms appear.
- Simple Explanation: ML identifies subtle correlations in data (e.g., rising lactate levels + low platelets = sepsis risk).

3. Error Reduction in High-Volume Testing
AI flags anomalies in results (e.g., mislabeled samples, outlier values) that humans might miss.
- Case Study: A UK lab reduced misdiagnoses by 30% after implementing AI quality-control tools.
Technical Terms Explained
- Machine Learning (ML): A type of AI that improves automatically through experience (e.g., analyzing 10,000 blood samples to “learn” normal vs. abnormal ranges).
- Neural Networks: Algorithms modeled after the human brain, used to recognize complex patterns (e.g., tumor shapes in MRI scans).
- Convolutional Neural Networks (CNNs): Specialized neural networks for image analysis.

Challenges to Consider
- Data Privacy: AI requires vast patient datasets — labs must comply with GDPR/HIPAA.
- Integration Costs: Upgrading legacy lab systems to AI-ready platforms can be expensive.
- Human Oversight: AI is a tool, not a replacement — lab professionals must validate results.
The Future of AI in Labs
- Instant Diagnostics: Portable AI devices (e.g., smartphone-based microscopes) for rural areas.
- Personalized Treatment Plans: AI combining genomic data with lab results to tailor therapies.
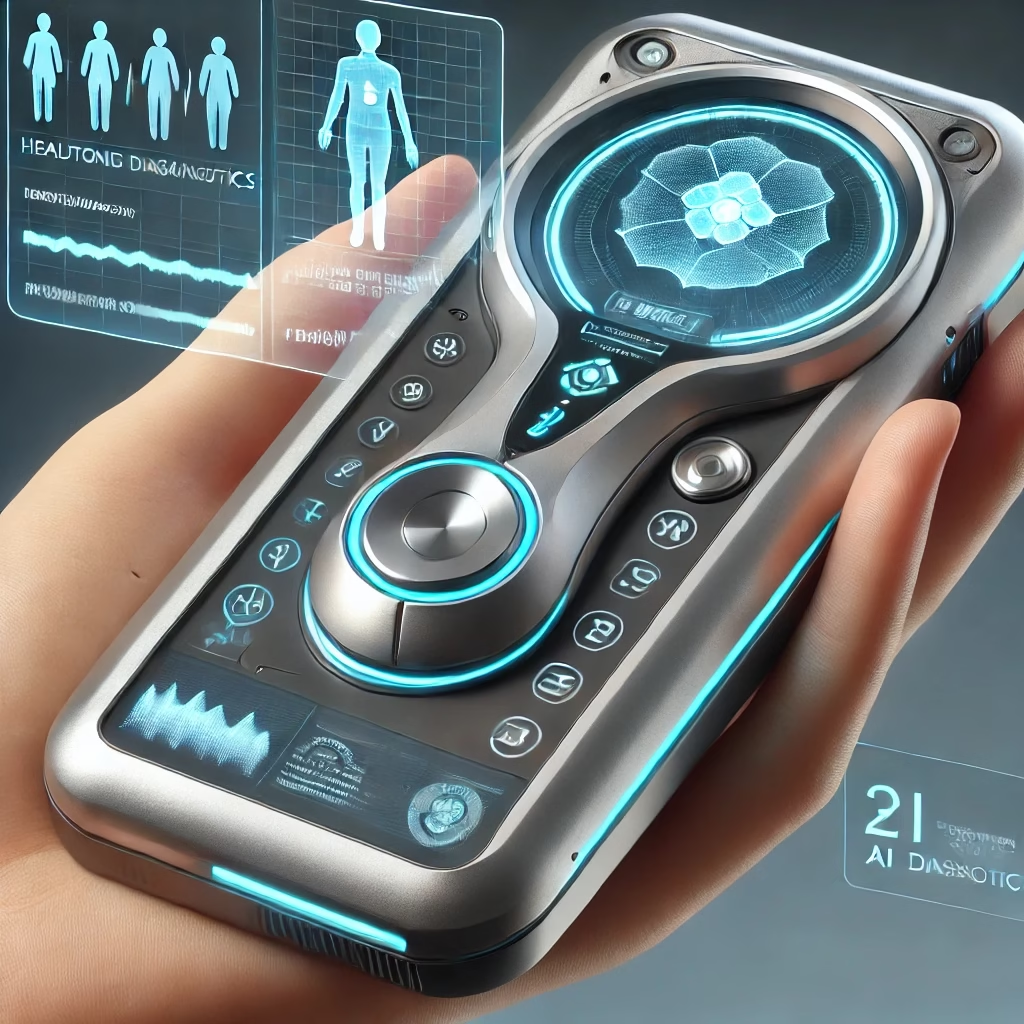
Conclusion
AI isn’t here to replace lab professionals — it’s here to amplify their expertise. By automating repetitive tasks and highlighting critical insights, AI lets scientists focus on what matters: patient care.
CTA: “How is your lab adapting to AI? Share your thoughts in the comments!”
References
Nature Journal. PathAI’s Histopathology Breakthroughs.
World Health Organization (2023). AI in Healthcare Report.
Copyrights Recieved for MLS GATE 2025 ©